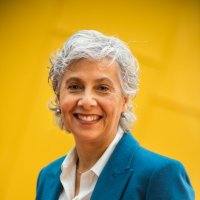
PI
Core/Dual
Una-May O'Reilly
To make our lives and systems more secure, Dr. Una-May O’Reilly’s research goals are to understand adversarial intelligence and to computationally model it with machine learning. Dr. O’Reilly hopes that by developing Artificial Adversarial Intelligence it will help reveal the dynamics of conflicting behavior and how adaptation drives it, leading to more secure systems.
Dr. O’Reilly, who holds a PhD from Carleton University in Ottawa, Canada and joined CSAIL in 1996, was drawn to this area of research when pursuing her highly regarded and award-winning work on evolutionary algorithms. These algorithms are a good fit to model the evolving arms race between tax avoidance and tax loophole remediation. They supported an approach to identifying vulnerabilities in a subsection of the Internal Revenue code while avoiding auditing, even under auditing co-adaptation. Now one of the main areas Dr. O’Reilly is investigating is cybersecurity and how to stop destructive and escalating arms races between cyber attack actors and cyber defenders.
Another area she is exploring is the security of software. Dr. O’Reilly, who is interested in understanding computer programs in general, conducts neuroscience experiments to reveal how humans read code and uses machine learning models to encode software. Her research also dives into deep learning techniques for program representations. Typically, code varies in length, obeys syntax, and expresses semantics. To automatically detect bugs or code malware, a computer-friendly representation of code that encompasses all of these properties is required. Such representations can be used by software enterprises in machine learning detectors and classification training.
Most recently Dr. O'Reilly, who is passionate about addressing climate change, is studying disinformation flows through social media platforms supported by computer and AI/ML technology that enables its amplification at very accelerated rates. In the context of climate disinformation, she investigates "organic" influence across social media, how a disinformation campaign expresses a narrative, and how disinformation is seeded and disseminated around critical events.
Dr. O’Reilly’s security-driven, adversarial-focused approach to AI and machine learning will lead to more secure systems, better program comprehension, and improved disinformation awareness.
BIO
Una-May is leader of the AnyScale Learning For All (ALFA) group at CSAIL. ALFA focuses on scalable machine learning, evolutionary algorithms, and frameworks for large scale knowledge mining, prediction and analytics. The group has projects in cybersecurity, healthcare, and online education.
Una-May was awarded the EvoStar Award for Outstanding Contribution of Evolutionary Computation in Europe in April, 2013. She is also is a Fellow of the International Society of Genetic and Evolutionary Computation, now ACM Sig-EVO.
Una-May co-founded ACM SigEVO in 2004. She serves as Vice-Chair of ACM SigEVO. In 2013 she inaugurated the Women in Evolutionary Computation group at GECCO.
Una-May served as chair of the largest international Evolutionary Computation Conference, GECCO, in 2005. She has served on the GECCO business committee, co-led the 2006 and 2009 Genetic Programming: Theory to Practice Workshops and co-chaired EuroGP, the largest conference devoted to Genetic Programming.
Una-May serves as the area editor for Data Analytics and Knowledge Discovery for Genetic Programming and Evolvable Machines (Kluwer), as editor for Evolutionary Computation (MIT Press), and as area editor for ACM Transactions on Evolutionary Learning and Optimization.
Una-May has a patent for an original genetic algorithm technique applicable to internet-based name suggestions.
Una-May holds a B.Sc. from the University of Calgary, and a M.C.S. and Ph.D. (1995) from Carleton University, Ottawa, Canada. She joined the Artificial Intelligence Laboratory, MIT as a Post-Doctoral Associate in 1996.
Related Links
Last updated Jan 11 '23