Project
Adversarial Cyber Security
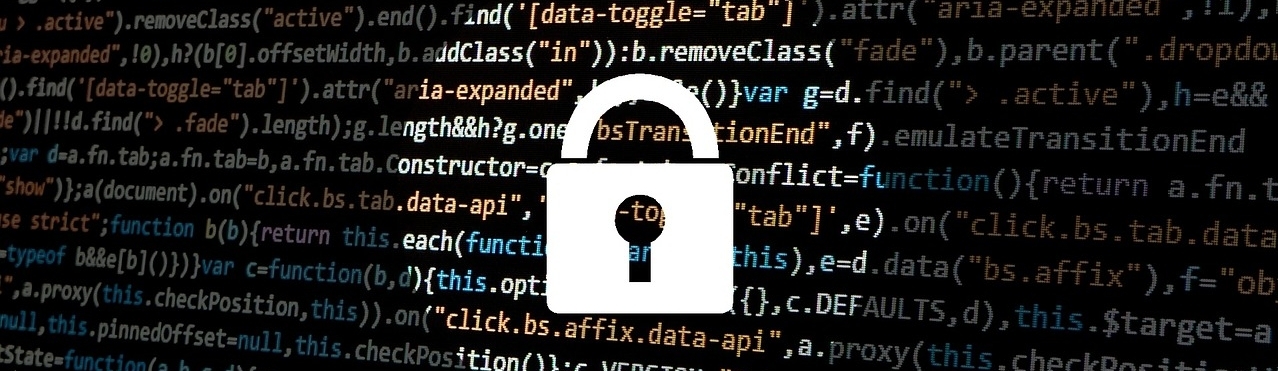
Cyberspace has become a competition ground occupied by intelligent, adaptive adversaries. Defenders and attackers engage in arms races as both sides take turns crafting new responses to each other’s actions. The arms races play out in multiple cyber arenas ranging from networks sustaining Denial of Service attacks, to compromised enterprise systems being profiled by internal reconnaissance, to anti-virus detectors encountering unanticipated malware. Current defenses are largely reactive -- each new attack typically requires identification, human response, and design intervention to prevent it. They are inadequate to address the ever increasing scale, severity and adaptive strategies of malicious parties. Our vision is autonomous cyber defenses that anticipate and take measures against counter attacks. Our technical approach frames a robust optimization problem where the objectives of the two sides conflict and the positive gains of one side imply negative outcomes for the other. The co-optimization problem is solved with co-evolutionary algorithms.
Rivals: More robust and resilient networks under extreme DDoS attacks
This research asks how simulating the adaptation of distributed denial of service attacks and exploiting the adaptability of advanced peer to peer networks can result in more robust and resilient designs. It is supported by the USA Defense Advanced Research Project Agency under the XD3 program.
ADHD: Adversarial Dynamics when Harnessing Deception
This research examines deceptive defenses that obscure a software defined network while entrapping and slowing down internal reconnaissance within the Advanced Persistent Threat kill chain.
The Arms Race of Anti-Virus Detection and Viruses
This research addresses the problem of anti-virus systems continually falling prey to slight perturbations of viruses they can detect.
STEALTH: Understanding the Relationship between Tax Non-Compliance and Tax Law
The project's goal is to develop technology that enables the discovery of non-compliant partnership transaction patterns.
Through the use of partnerships and other "flow-through entities", taxpayers underreported more than $91 billion of income annually between 2006 and 2009 (GAO-14-453), and the trend shows little sign of stopping. T
STEALTH (Simulating Tax Evasion And Law Through Heuristics) allows us to identify sequences of financial transactions around partnerships that accomplish the same economic purpose with differences in tax consequence. By applying a robust co-optimization and artificial intelligence modeling approach, it learns observables that indicate the presence of non-compliant behavior.
Related Links
Points of Contact
MIT Student inquiries
alfa-apply@csail.mit.edu
General inquiries
nicolem@csail.mit.edu
Last updated Apr 28 '20