Project
Using Muscle Signals to Lift Objects with Robots
When envisioning robot assistants of the future helping people around the house or in factories, they work as a team with their human partners as fluidly as if they were another person. Since the key to good teamwork is always good communication, we need a way to seamlessly convey desired goals and commands to our robot helpers. Moving towards such a goal, this project looks at the person's muscle activity to inform the robot about how it should move to best assist the person while they collaborate. By using the muscles already involved in performing the task, the robot could almost become like an extension of yourself that can be controlled intuitively.
This represents a step towards building a vocabulary for communicating with a robot assistant in a more natural way. As we continue to enrich this vocabulary, adding more continuous motion estimation as well as more gestures, the human and robot can accomplish more complex manipulations.
The system monitors biceps and triceps activity using non-invasive EMG sensors. By processing the biceps activity, it can roughly estimate when the person moves their hand up or down so the robot can roughly follow their motions. The person can then tense or relax their arm to control the robot without moving their own hand. In addition, they can make small up or down gestures for finer control over the robot. So rather than model the muscles, limbs, and task to accurately determining the person's pose and infer what the robot should do to help, the system puts the person in command to let them guide the joint behavior and accomplish the task.
MIT News article
The news article about this project can be found here
Publication at the ICRA 2019 conference
Abstract
Seamless communication of desired motions and goals is essential for enabling effective physical human-robot collaboration. In such cases, muscle activity measured via surface electromyography (EMG) can provide insight into a person's intentions while minimally distracting from the task. The presented system uses two muscle signals to create a control framework for team lifting tasks in which a human and robot lift an object together. A continuous setpoint algorithm uses biceps activity to estimate changes in the user's hand height, and also allows the user to explicitly adjust the robot by stiffening or relaxing their arm. In addition to this pipeline, a neural network trained only on previous users classifies biceps and triceps activity to detect up or down gestures on a rolling basis; this enables finer control over the robot and expands the feasible workspace. The resulting system is evaluated by 10 untrained subjects performing a variety of team lifting and assembly tasks with rigid and flexible objects.
Poster
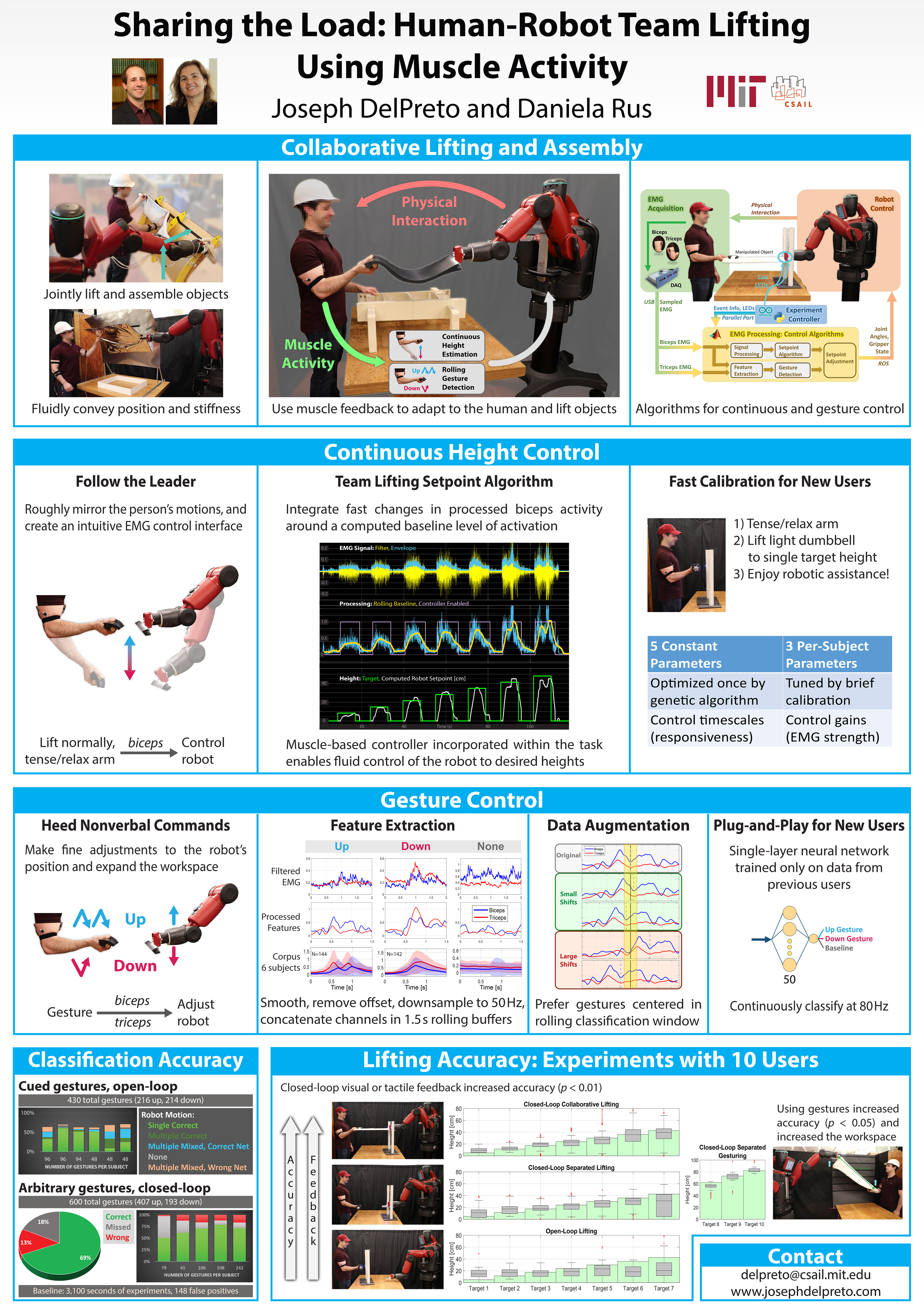
Video
Related Links
Contact us
If you would like to contact us about our work, please refer to our members below and reach out to one of the group leads directly.
Last updated Aug 13 '20