Project
Supervising Robots with Muscle and Brain Signals
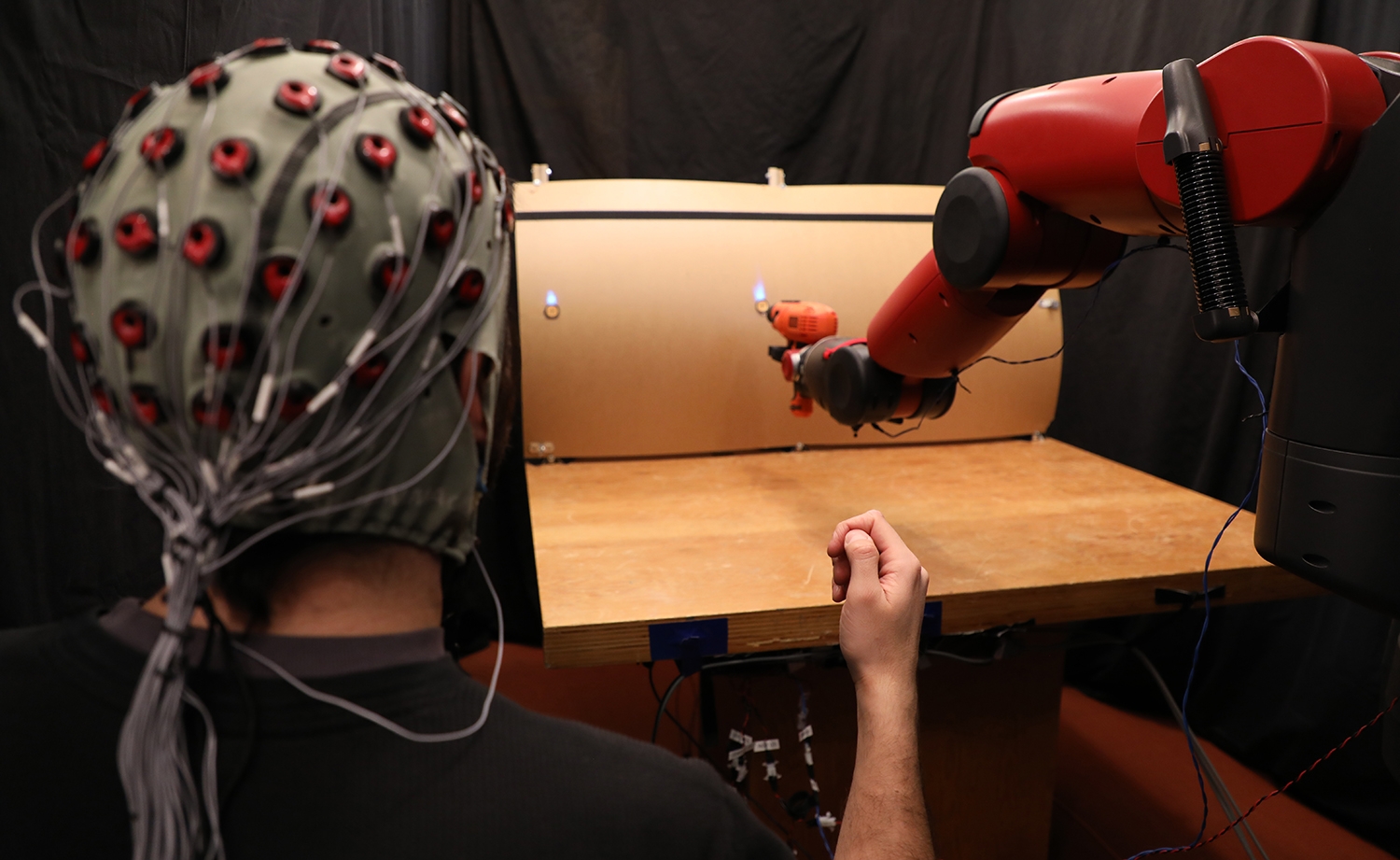
Robots are becoming more common in settings ranging from factories and labs to classrooms and homes, yet there's still somewhat of a language barrier when trying to communicate with them. Instead of writing code or learning specific keywords and new interfaces, we'd like to interact with robots the way we do with other people. This is especially important in safety-critical scenarios, where we want to detect and correct mistakes before they actually happen.
Taking a step towards this goal, we use brain and muscle signals that a person naturally generates to create a fast and intuitive interface for supervising a robot. In our experiments, the robot chooses from multiple targets for a mock drilling task. We process brain signals to detect whether the person thinks the robot is making a mistake, and we process muscle signals to detect when they gesture to the left or right; together, this allows the person to stop the robot immediately by just mentally evaluating its choices and then indicate the correct choice by scrolling through options with gestures.
- MIT News article
- Video (https://youtu.be/_Or8Lt3YtEA)
- Publication PDF: AURO 2020
- RSS Conference Poster
- More information on detecting robot mistakes using brain signals
Publication authors:
Joseph DelPreto, Andres F. Salazar-Gomez, Stephanie Gil, Ramin Hasani, Frank H. Guenther, and Daniela Rus
Publication abstract:
Effective human supervision of robots can be key for ensuring correct robot operation in a variety of potentially safety-critical scenarios. This paper takes a step towards fast and reliable human intervention in supervisory control tasks by combining two streams of human biosignals: muscle and brain activity acquired via EMG and EEG, respectively. It presents continuous classification of left and right hand-gestures using muscle signals, time-locked classification of error-related potentials using brain signals (unconsciously produced when observing an error), and a framework that combines these pipelines to detect and correct robot mistakes during multiple-choice tasks. The resulting hybrid system is evaluated in a "plug-and-play" fashion with 7 untrained subjects supervising an autonomous robot performing a target selection task. Offline analysis further explores the EMG classification performance, and investigates methods to select subsets of training data that may facilitate generalizable plug-and-play classifiers.
Publication PDF: available here
Related Links
Contact us
If you would like to contact us about our work, please refer to our members below and reach out to one of the group leads directly.
Last updated Aug 12 '20