Research Center
Center for Deployable Machine Learning (CDML)
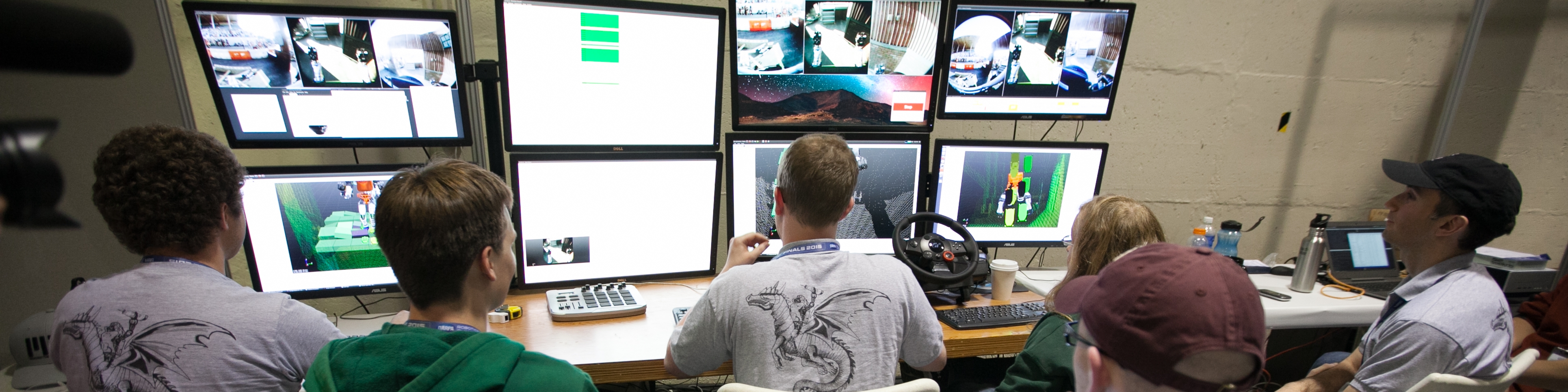
The impressive—often "super-human"—performance of state-of-the-art learning systems creates a major expectation that broad deployment of machine learning will revolutionize almost every aspect of our lives. However, fulfilling this expectation requires ML that is robust to a variety of random and adversarial corruptions, provides reliable decision-making, and is understandable and easy to work with for humans, even if they have no ML expertise. The goal of the MIT Center for Deployable Machine Learning (CDML) is to bring together the broad expertise and focused effort needed to build ML systems that are safe, robust, and reliable enough to be confidently and responsibly deployed in the real world.
Related Links
Contact us
If you would like to contact us about our work, please refer to our members below and reach out to one of the group leads directly.
Last updated Aug 02 '21