Project
PrivacyML - A Privacy Preserving Framework for Machine Learning
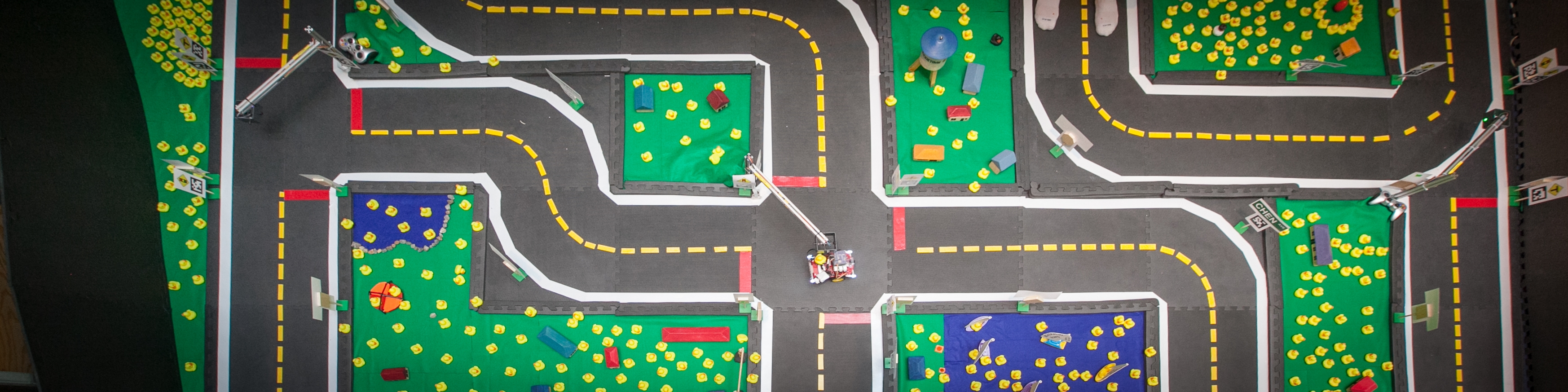
Enterprises usually provide strong controls to prevent external cyberattacks and inadvertent leakage of data to external entities. These controls usually focus on restricting unauthorized access. In the case where employees and data scientists have legitimate access to analyze and derive insights from the data, they are permitted access to all information about the customers of the enterprise including sensitive and private information. Though it is important to be being able to identify useful patterns of one’s customers for better customization and service, we do not believe that customers’ privacy must to be sacrificed to do so.
One approach is to develop privacy preserving versions of machine learning algorithms. However, this requires analysts to be intimately familiar with privacy and be constantly aware of it. Our approach is to develop a general framework that enforces privacy internally enabling different kinds of machine learning to be developed that are automatically privacy preserving. This decoupling of privacy preservation and machine learning based analysis is important because it reduces the additional burden of privacy protection. Our goal is to protect the data from analysts who want to analyze it for various purposes while still enabling its utility.
Contact us
If you would like to contact us about our work, please refer to our members below and reach out to one of the group leads directly.
Last updated Apr 30 '24