Project
The Car Can Explain!
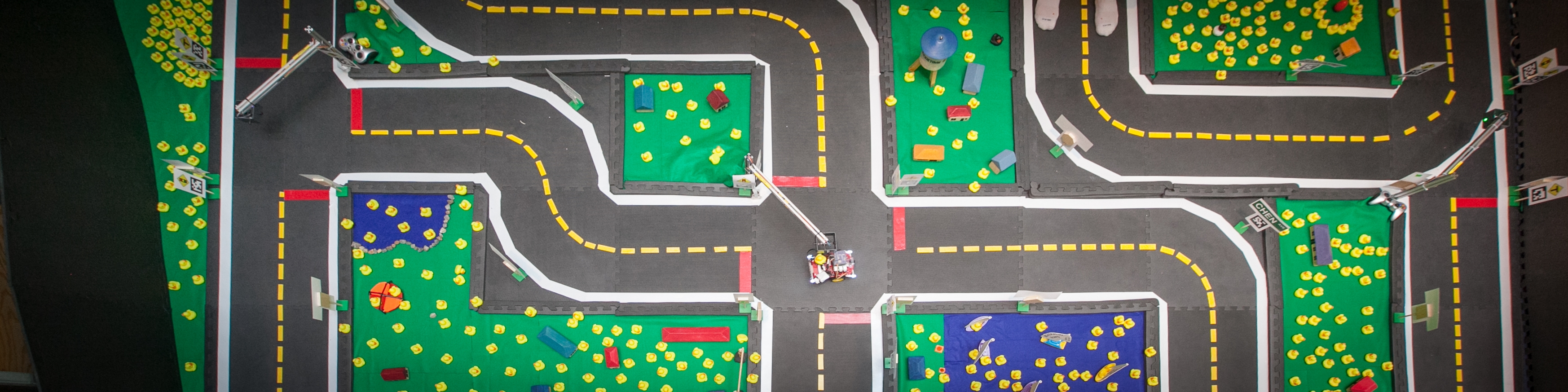
When humans and autonomous systems share control of a vehicle, there will be some explaining to do. When the autonomous system takes over suddenly, the driver will ask why. When an accident happens in a car that is co-driven by a person and a machine, police officials, insurance companies, and the people who are harmed will want to know who or what is accountable for the accident. Control systems in the vehicle should be able to give an accurate unambiguous accounting of the events. Explanations will have to be simple enough for users to understand even when subject to cognitive distractions. At the same time, given the need for legal accountability and technical integrity these systems will have to support their basic explanations with rigorous and reliable detail. In the case of hybrid human-machine systems, we will want to know how the human and mechanical parts contributed to final results such as accidents or other unwanted behaviors.The ability to provide coherent explanations of complex behavior is also important in the design and debugging of such systems, and it is essential to give us all confidence in the competence and integrity of our automatic helpers. But the mechanisms of merging measurements with qualitative models can also enable more sophisticated control strategies than are currently feasible. Our research explores the development of methodology and supporting technology for combining qualitative and semi-quantitative models with measured data to produce concise, understandable symbolic explanations of actions taken by a system built out of many parts (including the human operator).
Group
Decentralized Information Group (DIG)Communities
Cognitive AI Community of Research Computing & Society Community of ResearchRelated Links
Contact us
If you would like to contact us about our work, please refer to our members below and reach out to one of the group leads directly.
Last updated Oct 06 '20