Project
Understanding Convergence of Iterative Algorithms
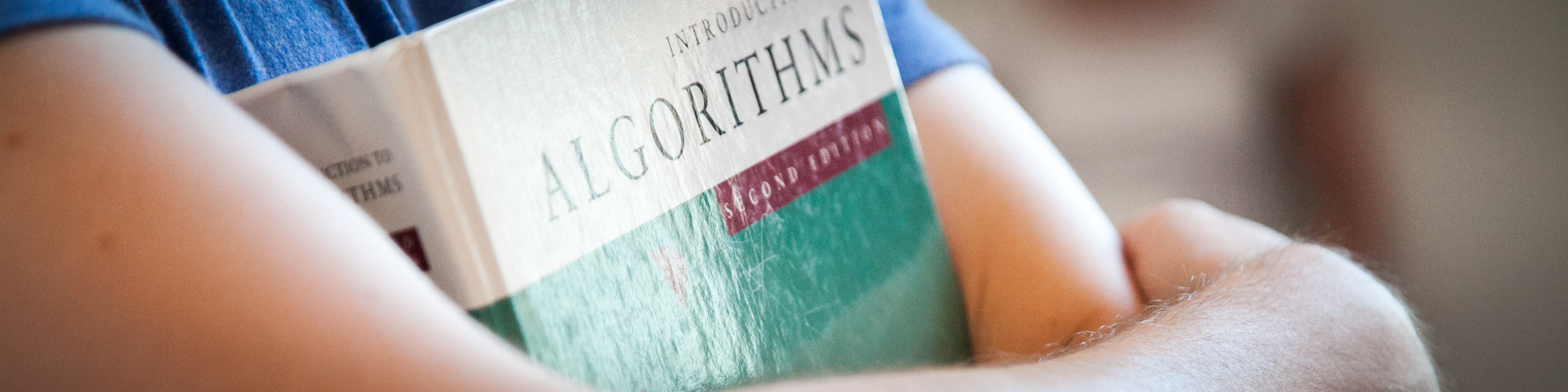
The increasing interest of machine learning, on non-convex problems, has made non-convex optimization one of the most challenging areas of our days. Despite of this increasing interest too little is known from a theoretical point of view. The purpose of this project is to make a step in the direction of investigating a rich enough toolbox, to be able to analyze non-convex optimization. Contraction maps and Banach’s Fixed Point Theorem are very important tools for bounding the running time of a big class of iterative algorithms used to solve non-convex problems. We explore how generally we can apply Banach’s fixed point theorem to establish the convergence of iterative methods when pairing it with carefully designed metrics. Our first result is a strong converse of Banach’s theorem, showing that it is a universal analysis tool for establishing uniqueness of fixed points and convergence of iterative maps to a unique solution. We also turn to applications proving global convergence guarantees for one of the most celebrated inference algorithms in Statistics, the EM algorithm. Proposed in the 70’s, the EM algorithm is an iterative method for maximum likelihood estimation whose behavior has vastly remained elusive. We show that it converges to the true optimum for balanced mixtures of two Gaussians by introducing another general tool for analysis of iterative algorithms which we call the sensitivity method. Since as we explained too little is known in this direction there are a lot of very interesting open problems that can both generalize the above results and produce new ideas and techniques.
Group
Algorithms GroupContact us
If you would like to contact us about our work, please refer to our members below and reach out to one of the group leads directly.
Last updated Oct 18 '17