Project
Robust Optimization in Machine Learning and Data Mining
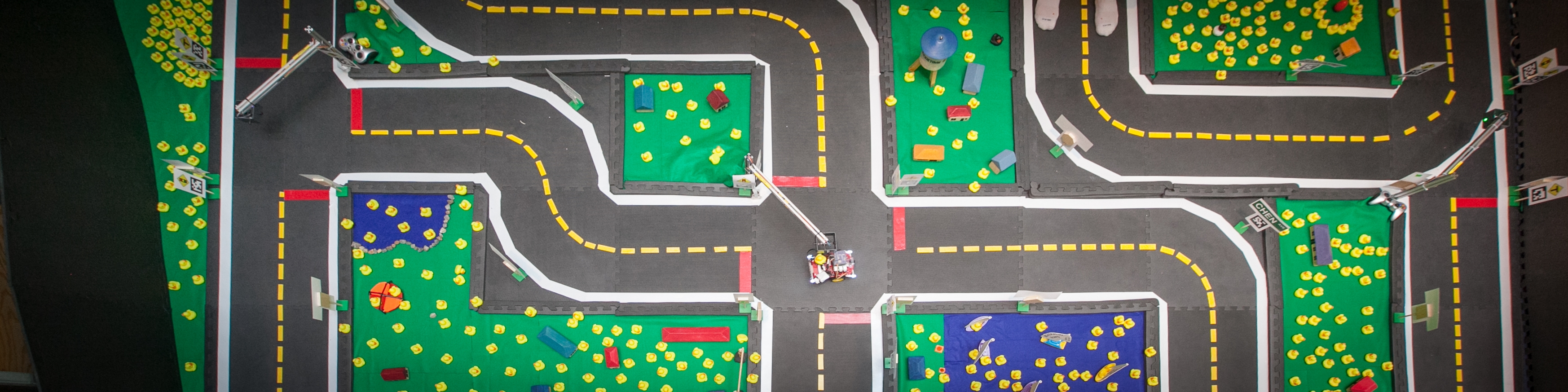
Many optimization problems in machine learning and data mining, such as summarization, budget allocation, and influence maximization, rely on parameters that are learned from noisy data. Oftentimes in academic literature, the optimization problem setups that exist are not realistic in the sense that they are too clean and offer more information than is typically provided in the real world. Our project aims to mathematically account for noisy data and randomness in realistic settings, so that a machine-learning system can make a decision that works well even under such uncertain conditions.
For example, if you are working with a baseball model that has a particular assumption such as the batting average of a player on the other team, and the player hasn't played many games, you probably don't have a very good idea of what the player's batting average really is. Even if you are accounting for all of the randomness of the game itself, you may get the batting average wrong and therefore make a suboptimal decision for maximizing how many games your team wins.
But it can be challenging to capture the ways in which a model or dataset might be noisy or perturbed, especially in mathematical terms that lead to efficient algorithms. There is a trade-off between how precisely you can narrow down how your model is wrong, vs. the kinds of phrasings that can be optimized efficiently. Oftentimes, you do not have perfect access to the randomness, and, in addition, the functions you are optimizing might be very complicated. The main focus of our research is to simultaneously address these two issues in different settings.
We are creating a toolkit containing optimization algorithms that help you make good decisions efficiently, in a wide variety of settings where the data or models might have some inaccuracies. Our goal is that a practitioner should be able to write down possible issues that may arise within a dataset, and we should be able to provide an algorithm that helps the practitioner make a decision or learn a model that works well, explicitly guarding against the listed concerns. Ultimately, we are striving to have algorithms that contribute to a decision that works well even if the predicted problems happen.
By carefully exploiting the mathematical structure of such problems, we are still able to develop robust optimization algorithms with theoretical guarantees, with the end goal of practical, efficient, and reliable algorithms that perform well in the face of uncertainty.
Group
Machine LearningContact us
If you would like to contact us about our work, please refer to our members below and reach out to one of the group leads directly.
Last updated Apr 28 '20