Project
Reliable and Robust Machine Learning
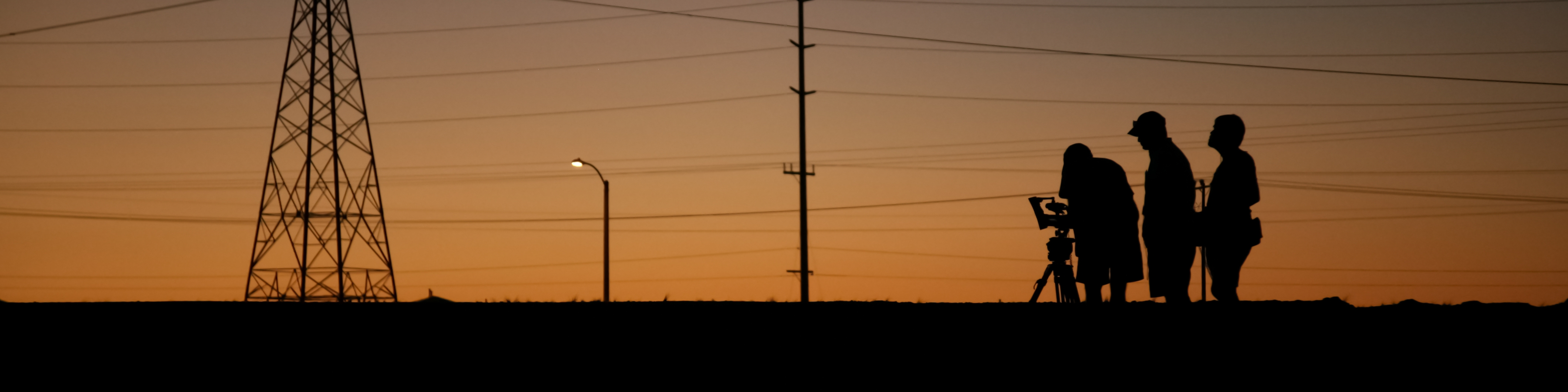
Machine learning has made breakthrough advances in computer vision, language translation, and many other tasks. The outstanding performance our current ML toolkit achieves in benchmarks suggests it performs these tasks at a truly human level. The truth, however, turns out to be more nuanced.
The moment we move beyond the clean and controlled/simulated settings in which ML has been largely developed in so far (and the setting it was mostly targeting during this development), the corresponding average-case/“proof of concept” mindset turns out to be grossly inadequate. The high-stakes nature of the real-world deployment of ML makes worst-case—rather than average-case—performance the chief design goal. That is, we need our ML tools to be robust to the variety of corruptions that abound in real-world contexts, whether benign or malicious in nature. It is also necessary to have a fine-grained grasp of the biases learned by our models and the impact of employing them in decision making. Finally, to be truly useful, our ML tools need to be understandable to humans and easy to work with even for users with no ML expertise.
The current ML toolkit and, in particular, deep neural network models largely fail catastrophically with respect to the above criteria. We are thus working on developing understanding and methodology that will enable us to change this.
Group
Algorithms GroupContact us
If you would like to contact us about our work, please refer to our members below and reach out to one of the group leads directly.
Last updated Feb 10 '20