Project
Optimal transport for statistics and machine learning
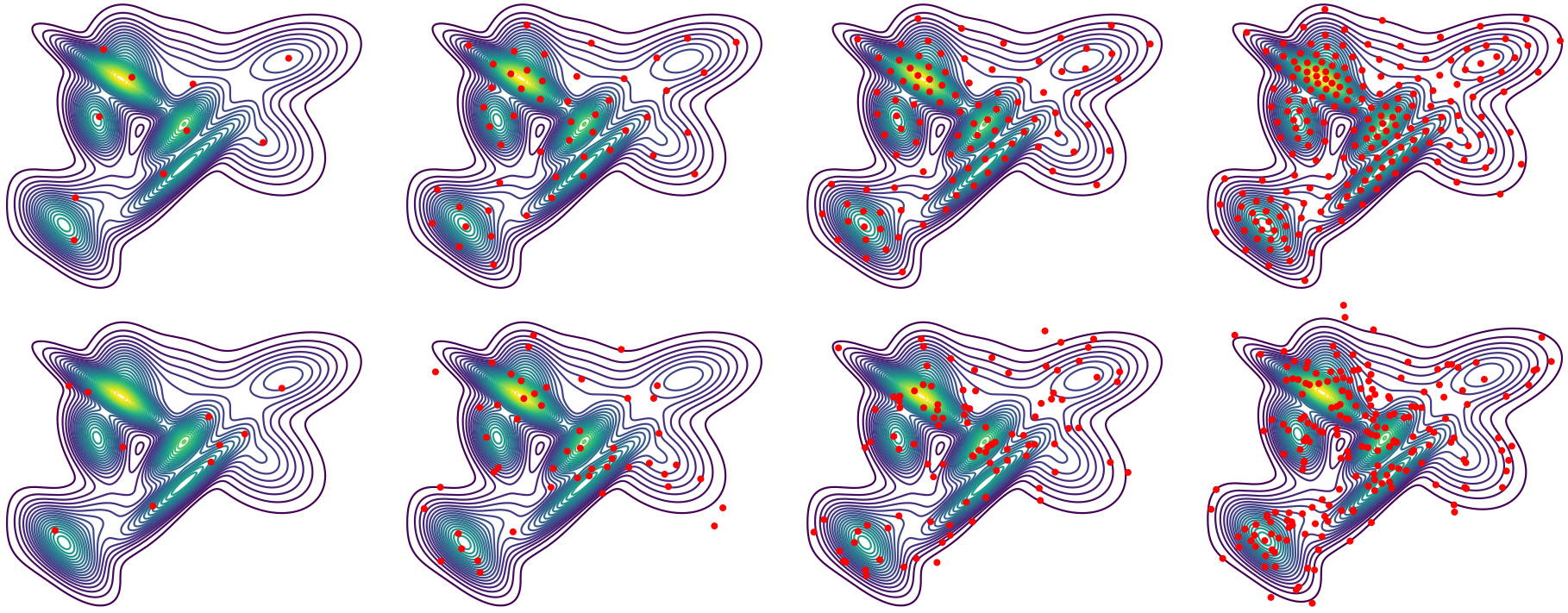
Optimal transport (OT) lifts ideas from classical geometry to probability distributions, providing a means for geometric computation on uncertain data. The key computational challenge in bringing OT to applications, however, is to develop efficient algorithms for solving OT problems on large-scale datasets, high-dimensional probability distributions, and in the context of larger machine learning pipelines. Our team is advancing the forefront of applied OT by expanding its scope and scalability, transitioning OT from theory to practice.
Our numerical OT algorithms leverage the structure of the domain to accelerate the most critical and common applications, including approximate OT on meshes and grids for graphics, stochastic techniques for learning, graph algorithms for operations, and finite elements for scientific computing. This new machinery enables countless applications for OT, from surface correspondence, audio effects, and vector field design in graphics/media to Bayesian inference, document retrieval, label propagation, and representation learning in machine learning.
Related Links
Contact us
If you would like to contact us about our work, please refer to our members below and reach out to one of the group leads directly.
Last updated Jan 25 '20