Project
KPart: A novel technique for partitioning shared caches
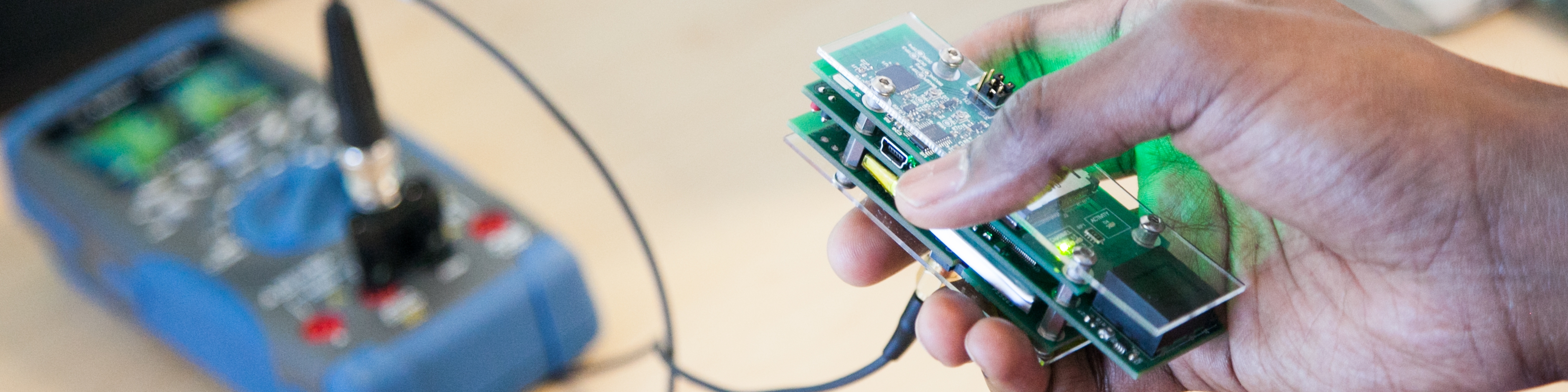
In this project we investigate novel techniques for partitioning shared resources among co-running applications in multicore systems.
Cache partitioning is now available in commercial hardware. In theory, software can leverage cache partitioning to use the last-level cache better and improve performance. In practice, however, current systems implement way-partitioning, which offers a limited number of partitions and often hurts performance. These limitations squander the performance potential of smart cache management.
KPart is a hybrid cache partitioning-sharing technique that sidesteps the limitations of way-partitioning and unlocks significant performance on current systems. KPart using machine learning clustering techniques to group co-running applications into clusters, then partitions the cache among these clusters. An evaluation of KPart on a real system shows that this approach can improve throughput by 24% on average (up to 79%) on an Intel Broadwell-D system.
Source code: https://github.com/Nosayba/kpart
Project Members
CSAIL Members: Nosayba El-Sayed, Anurag Mukkara, Po-An Tsai, Daniel Sanchez (PI)
Non-CSAIL Members: Harshad Kasture (Oracle), Xiaosong Ma (Qatar Computing Research Institute, HBKU)
Related Links
Contact us
If you would like to contact us about our work, please refer to our members below and reach out to one of the group leads directly.
Last updated Feb 26 '18