Project
An Interlock for Self Driving Cars
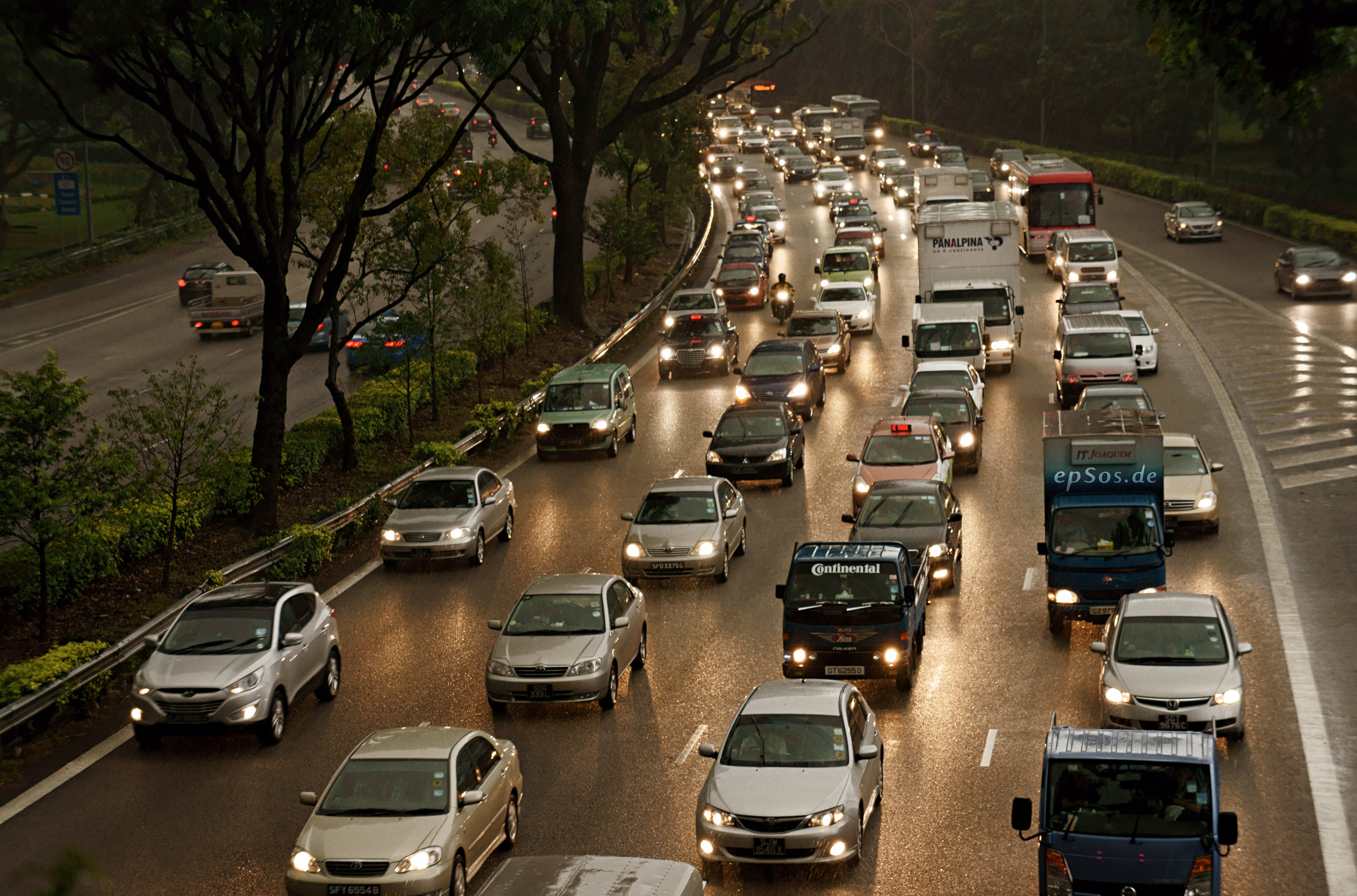
Traditional solutions for ensuring reliability in self-driving cars use sensor monitors that take in sensor data directly, and these monitors have to interpret the sensor data and compare their interpretations against the main controller. The safety controller, or interlock, monitors the environment and intervenes in plenty of time when an accident is imminent. In practice, however, the traditional interlock theory only goes so far, often failing to intervene or intervening unnecessarily. How do we ensure that the interlock intervenes when it should in such a complex system as an autonomous vehicle?
Our project takes a new approach that equips self-driving cars with tools for perception and situational awareness that are just as sophisticated as those of the main controller. We do this by means of certified control. Certified control is a new architecture for autonomous cars that offers the possibility of a small, verifiable trusted base without preventing the use of complex machine-learning algorithms for perception and control.
The key idea is to exploit the classic gap between the high cost of finding a solution to a problem and the much lower cost of checking that solution. The main controller plays the role of the solver, analyzing the scene and determining an appropriate next step, and the certifier plays the role of the checker, ensuring that the proposed step is safe.
To make this check possible, the main controller constructs a certificate that captures its analysis of the situation along with the proposed action. The main controller is thus excluded from the trusted base: when it works correctly, the certifier endorses its commands; and when it fails, the certifier will reject the commands and a simpler controller will bring the car to a safe stop. We have designed an architecture that embodies this idea, and demonstrated it in simulation and in a racecar.
For example, if the main controller thinks that you could drive ahead, it has to provide LiDAR scans indicating that there is indeed no obstacle ahead of the car. This sensor data has to be signed by the sensor providing it so that the controller cannot fake any data that would prove an unsafe situation to be safe.
This method goes beyond traditional runtime monitors that try to make proofs to show the safety of the control system, in that the checks that certified control maintains on the system are simple and easy to understand, as well as created by the designer long before the car is on the road. With certified control, the designer of the vehicle’s system can choose the level of safety and checks they would like to make, empowering the designer to not only make the vehicle safe but also prove to the public that the car is safe enough to be adopted.
This approach pushes us closer to making vehicle systems and algorithms in such a way to guarantee they operate as good as or better than human drivers under many different kinds of conditions, and convincing the general public and regulators that self-driving cars can do this reliably.
So far, we have explored two examples of complex solving. The first involves finding lane lines using visual analysis. In this case, the certificate includes a signed camera image and a mathematical specification of purported lane lines. The checker ensures that the lane lines obey the standard conventions (i.e., being parallel and the right distance apart), and that they match the markings on the road, as given in the camera image. We have tested this approach on sample videos from the Open Pilot project, and shown that we are able to catch cases in which lane detection produces bad results.
The second involves filtering LiDAR data to remove spurious reflections from snow. The main controller applies an outlier-detection algorithm to remove points from the LiDAR cloud that correspond to snowflakes, and selects from what remains a set of points that cover the lane ahead with enough density to ensure that no obstacle larger than a certain size can be present. We have demonstrated this approach using a 3D Velodyne LiDAR mounted on our racecar, and have shown that the certifier correctly allows the case in which the car faces simulated snow, but rejects a certificate in which the filtering removes obstacles that are too large (such as some cables dangling in front of the car).
Currently, we are also testing in CARLA, an open-source self-driving car simulator, and migrating the existing code for the checkers we have developed into CARLA, with successful testing in CARLA against our LiDAR interlock. Our focus now is on LiDAR, because there are already rich algorithms that people use to detect features in LiDAR scans, and being able to verify those will contribute a lot to self-driving car technology. In addition, every LiDAR data point gives some physical information about the real world, which gives it an advantage over individual pixels in a camera feed.
This project is part of a collaboration between CSAIL and the Toyota Research Institute, and is funded in part by Toyota. A patent describing certified control has been filed.
For more information, see a workshop paper from DARS 2019 and a recent talk.
Group
Software Design Group Computer-Aided ProgrammingCommunities
Vertical AI Community of Research Human-Computer Interaction Community of ResearchRelated Links
Sponsored By
How to contact us
Contact us
If you would like to contact us about our work, please refer to our members below and reach out to one of the group leads directly.
Last updated Apr 07 '22