Project
Detecting Voice Misuse to Diagnose Disorders
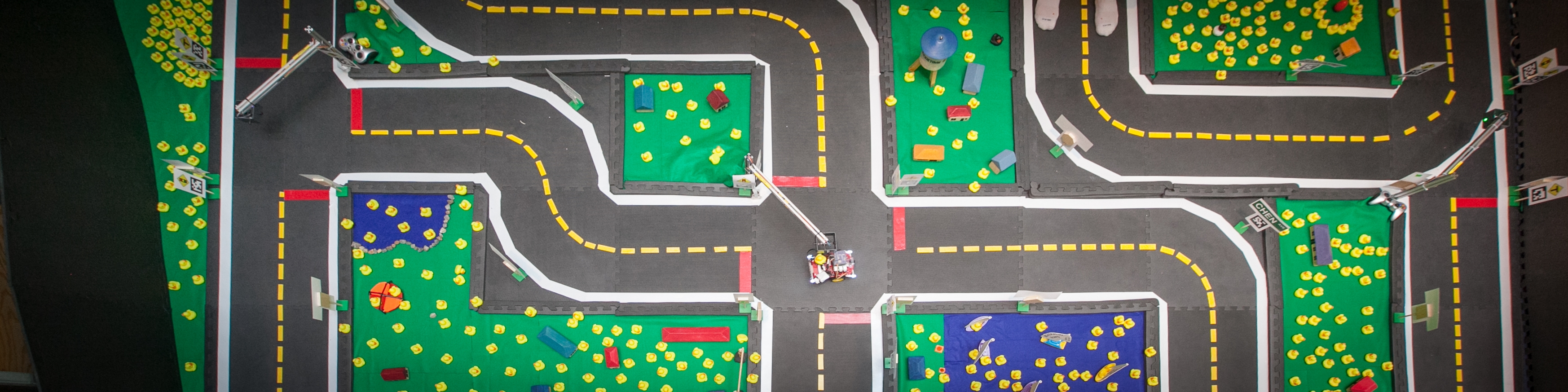
Our work focuses on using technology to help detect vocal misuse, patterns, and pathologies. In this project, we collected accelerometer data from a wearable device worn around the neck, developed by researchers at the MGH Voice Center. We created a learning algorithm that examines what vocal cord movements are prominent in subjects with disorders, using unsupervised learning, where data is unlabeled at the instance level. By analyzing more than 110 million “glottal pulses” (representing one opening and closing of the vocal folds) and comparing clusters of pulses, we detected significant differences between patients and controls.
Marzyeh Ghassemi
Last updated Dec 06 '17
Research Areas
Impact Areas
Members
Publications
M. Ghassemi, P. Szolovits.
“Predicting early psychiatric readmission with natural language processing of narrative discharge summaries.”
Translational Psychiatry – Nature 2016.
M. Ghassemi, T. Naumann.
“A Multivariate Timeseries Modeling Approach to Severity of Illness Assessment and Forecasting in ICU with Sparse, Heterogeneous Clinical Data.”
AAAI 2015.
M. Ghassemi, P. Szolovits.
“Unfolding Physiological State: Mortality Modelling in Intensive Care Units.”
KDD 2014.