Project
Deep Inverse Planning
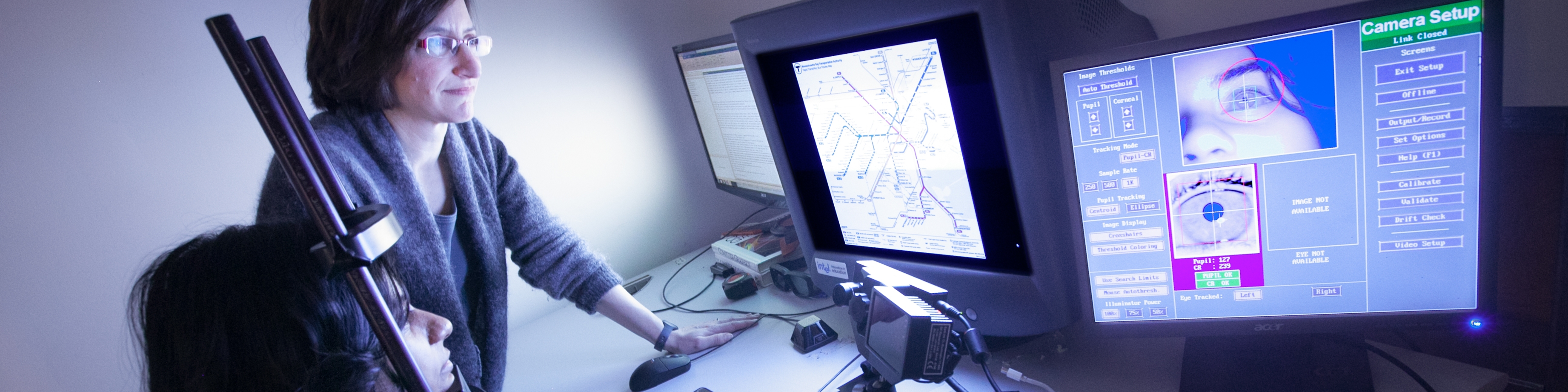
In the context of planning problems, observing human demonstrations allows a system to efficiently learn a policy (mapping of states to actions) through methods such as inverse optimal control (IOC). However, many complex problems are embedded within decision processes which require exceedingly high-dimensional representations. Unfortunately, traditional approaches to IOC become intractable when faced with this high-dimensionality. Recent work in deep reinforcement learning addresses this limitation by learning neural network parameters for estimating the expected value of actions from a given state. Once trained, the network efficiently maps raw visual input to a model free policy. However, this process fails when feedback from the environment is not available and the reward/cost function requires a non-linear high-dimensional parameterization. We propose extending recent work in deep reinforcement learning to the inverse planning setting for efficiently learning complex behaviors from high-dimensional data without the need for environment feedback (rewards, coordinates, etc.) or low-dimensional reward/cost functions.
Group
Vision GroupContact us
If you would like to contact us about our work, please refer to our members below and reach out to one of the group leads directly.
Last updated Jun 22 '17