Collapse-Proof Non-Contrastive Self-Supervised Learning
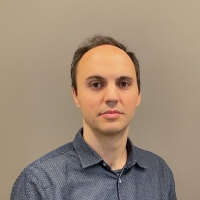
Speaker
Host
Abstract: Self-supervised learning (SSL) has unlocked the potential of learning general-purpose representations from large amounts of unlabeled data. Despite its successes, important challenges remain, hindering the applicability and democratisation of SSL. One such challenge is the presence of failure modes occurring during the training of SSL models. In this talk, we aim to distill the essential principles to guarantee the avoidance of known collapses. We present a principled and simplified design of the projector and loss function for non-contrastive SSL based on hyperdimensional computing. We theoretically demonstrate that this design introduces an inductive bias that encourages representations to be simultaneously decorrelated and clustered, without explicitly enforcing these properties. This bias provably enhances generalization and suffices to avoid known training failure modes, such as representation, dimensional, cluster, and intracluster collapses. We validate our theoretical findings on image datasets, including SVHN, CIFAR-10, CIFAR-100, and ImageNet-100. Our approach effectively combines the strengths of feature decorrelation and cluster-based SSL methods, overcoming training failure modes while achieving strong generalization in clustering and linear classification tasks.
Bio: Emanuele Sansone is a Postdoctoral Fellow jointly affiliated with MIT (CSAIL) and KU Leuven (ESAT). His research interests lie at the intersection between unsupervised learning and mathematical logic. His research ambition is to empower machines with the capability to acquire and discover knowledge from data in an autonomous manner. He was recently awarded the Marie Curie Global Fellowship for the program titled “Discovering the World through Unsupervised Statistical Relational Learning”.